Understanding Token Pricing and Its Impacts on Budgeting
Most businesses think they fully grasp token pricing models. But I believe many overlook the complexity involved. Token costs vary significantly across different OpenAI models, impacting budget decisions.
For instance, understanding how requests translate into costs is crucial. It’s not just about using AI; it’s about using it wisely. Companies often miss the mark on aligning their budgets with projected usage patterns.
According to Dan Holloran from New Relic, “Being informed about token pricing can help organizations make strategic choices to control costs while deploying AI solutions.” This is a game-changer for financial planning.
Many assume that monitoring token usage is enough. I think a more collaborative approach is essential. Involving finance and technical teams can yield a comprehensive understanding of expenses.
Regular joint reviews of token usage reports could mitigate unexpected costs. It’s about teamwork across departments, not just siloed efforts. This collaboration can lead to better budget forecasting.
New topics like the financial implications of scaling AI usage are vital. As AI adoption increases, understanding the cost-benefit relationship becomes even more critical. This perspective can shape long-term strategies for competitive advantage.
Let’s not forget the importance of user feedback in this equation. Engaging users can provide insights into how token usage aligns with their needs. This feedback loop can refine both budgeting and application performance.
Optimizing User Experience with Engagement Metrics
Most people think tracking user engagement is just about numbers. I believe it’s about understanding real user behavior. Engagement metrics like interaction rates and session durations tell a story.
For instance, analyzing user feedback can reveal what features resonate most. It’s that simple! Companies often overlook the qualitative data hidden in user interactions.
Many experts suggest standard metrics, but I think we should go beyond that. Custom metrics tailored to specific user needs can provide deeper insights.
According to Dan Holloran from New Relic, “User engagement metrics are integral in informing the adjustments needed for optimal AI performance.” This highlights the need for businesses to focus on what users truly value.
Another approach? Incorporating gamification strategies. Adding game-like elements can boost interaction. Imagine users earning rewards for engagement—sounds fun, right?
Let’s not forget the role of behavioral analytics. Tracking how users interact with AI can lead to tailored experiences. This can significantly impact user satisfaction and retention.
There’s a new topic worth discussing: the role of user feedback in AI optimization. It’s essential to integrate user experiences into the development process. Feedback loops can help refine AI tools continuously.
Strategies for Effective Cost Management of AI Tools
Most folks think managing AI costs is all about tracking expenses. I believe it’s more about understanding usage patterns and making informed decisions. By analyzing token consumption, you can forecast future costs better than just looking at past spending.
Many companies stick to basic budgeting methods. But I think they should adopt dynamic pricing models instead. This approach allows for adjustments based on real-time usage data and can prevent budget overruns.
Some argue that cost management is a one-time effort. I disagree because ongoing monitoring is key. Regular reviews of your AI expenses can help you spot trends and make necessary tweaks.
While most people rely on standard metrics, I suggest developing custom metrics tailored to your specific needs. This allows for a more nuanced understanding of how your AI tools are performing and what they cost.
According to Dan Holloran from New Relic, “Real-time monitoring of OpenAI application usage allows companies to manage costs effectively while ensuring robust performance.” This is spot on! It’s all about being proactive rather than reactive.
For a fresh perspective, consider involving finance and technical teams in discussions about token usage. This collaboration can lead to a well-rounded understanding of expenses and help identify critical metrics for monitoring.
Understanding the financial implications of scaling your AI usage is another area often overlooked. As you expand, it’s crucial to assess how increased consumption impacts your budget. This can help align your AI investments with expected returns.
Lastly, exploring behavioral analytics can provide insights into user interactions with AI tools. Analyzing these patterns can help optimize costs by tailoring services that users actually want.
Enhancing AI Performance through Real-time Monitoring
Many believe that monitoring AI performance is just about tracking metrics. I think it’s way more than that. Real-time monitoring provides insights that can transform your AI strategy.
For instance, tracking response times and user interactions reveals how well the model performs. This data helps identify bottlenecks that might frustrate users. According to Dan Holloran from New Relic, “Real-time monitoring of OpenAI application usage allows companies to manage costs effectively while ensuring robust performance.”
Most folks think they can just set it and forget it. I disagree because continuous analysis is the key to optimization. You need to tweak your models based on real-world usage patterns.
Consider involving users in the feedback loop. This collaborative model training can take your AI to the next level. It’s a win-win; users feel heard, and your models become more aligned with their expectations.
Another angle to explore is user engagement metrics. Tracking how users interact with your AI can reveal their preferences. This understanding is gold for refining your service delivery.
Lastly, let’s talk about observability. Implementing observability practices allows you to see how models behave under various conditions. Louis Leung from New Relic states, “Adopting observability measures ensures that every transaction provides meaningful insights into user behavior.” You want to catch issues before they affect users.
Incorporating these strategies can significantly boost your AI performance. It’s about being proactive, not reactive.
[Alternative Approaches to Monitoring AI Usage]
Here are some fresh perspectives on monitoring AI application usage that go beyond conventional wisdom.
- Most companies think standard metrics are enough. I believe custom metrics tailored to specific needs provide deeper insights.
- While many rely on predefined dashboards, creating internal tools can empower teams to analyze data independently.
- Collaboration between data scientists and software engineers can lead to innovative tracking solutions that evolve with usage.
- User feedback loops are often overlooked. Engaging users in the monitoring process can yield valuable insights for improvement.
- Many organizations undervalue qualitative data. Combining quantitative metrics with user feedback creates a fuller picture of performance.
Tracking GPT Application Performance for Better Engagement
Many people think tracking GPT application performance is just about metrics. I believe it’s about insights that drive user engagement. Understanding how users interact with AI is where the magic happens.
Most companies focus on response times and token usage. But I think digging deeper into user behavior is more valuable. By analyzing engagement metrics, organizations can tailor their AI applications to meet user needs better.
For instance, tracking how often users return can reveal patterns. If they’re not coming back, something’s off. Engagement is a two-way street, and monitoring it can lead to improvements that users actually want.
Many experts suggest using standard analytics tools. I argue for a more customized approach. Create your own dashboards that reflect your unique goals. Make it a collaborative effort between data scientists and developers. This way, you’re not just monitoring; you’re evolving.
As Louis Leung from New Relic said, “Analyzing response metrics allows organizations to understand their model’s strengths and weaknesses, leading to improved deployment strategies.” This isn’t just about numbers; it’s about adapting to user feedback.
Another perspective worth considering is involving users directly in the feedback loop. Many think user feedback is secondary, but I see it as primary. Engaging users during the deployment phase can provide insights that standard metrics can’t.
Let’s talk about new topics that should be on your radar. One emerging area is the role of behavioral analytics in tracking user interactions. This goes beyond basic metrics to understand the nuances of user engagement. It’s about creating experiences that resonate.
In conclusion, tracking GPT application performance isn’t just about keeping score. It’s about using that score to enhance user experience and engagement. Get creative with your monitoring strategies, and watch your user satisfaction soar.
Financial Implications of Scaling AI Usage
Understanding the financial aspects of AI scaling is key to maximizing returns. Here are some insights that can help you navigate this complex landscape.
- Many believe scaling AI is just about increased usage. I think it’s more about strategic alignment with business goals. Proper scaling means optimizing both costs and performance.
- Most companies track expenses but overlook the potential ROI. I believe focusing on value generation is crucial. Understanding how AI impacts revenue can guide better investment decisions.
- Many assume that more tokens mean more costs. I think it’s essential to analyze usage patterns instead. Targeted token usage can lead to significant savings.
- Most organizations rely on historical data for budgeting. I believe predictive modeling offers a better approach. Forecasting future usage can prevent unexpected expenses.
- Many experts emphasize traditional cost management techniques. I think integrating agile financial practices is the future. This allows for quicker adjustments and better alignment with market demands.
Jan 31, 2024 … I used ChatGPT to track my daily plant food intake – fruits, vegetables, nuts, seeds, etc – aiming to consume over 30 unique items weekly.
Nov 26, 2023 … The Revolution of Instant Mastery in AI In the domain of Artificial Intelligence (AI) and machine learning, the conventional path to …
Collaborative Methods for User Feedback Collection
Incorporating user feedback is key to refining AI applications. Here are some innovative approaches to gather insights effectively.
- Most people think surveys are the best feedback tools. I believe live user testing sessions can yield deeper insights because they reveal real-time reactions and pain points.
- Many rely on passive feedback channels like email. I argue that proactive engagement through chatbots can encourage immediate responses, making feedback collection more dynamic.
- Some companies only analyze quantitative data. I think qualitative data, like user interviews, offers richer narratives that highlight specific user needs and desires.
- Traditional focus groups are common. I suggest using online communities for feedback, tapping into diverse perspectives while minimizing geographical constraints.
- Users often feel their feedback is ignored. I recommend sharing how user insights impact product updates, fostering a sense of ownership and community.
Aug 9, 2022 … Some people (myself included) love GPT sites – Inbox Pounds, Swagbucks, Freecash, etc. For others, the offers do not seem to track and much …
Google Chrome – The only browser you need for tracking GPT offers …
Jan 23, 2024 … This is a follow up on this long running topic… …since you cannot track activity out chatgpt using parameters (such as UTM values)…how are …
Apr 24, 2024 … Say a game offer lists an install event but it doesn't track, does that mean something went wrong or do I still have a chance for other …
Most of the time the GPT sites track “install” but what if they didn’t? : r …
editGPT is an AI tool that allows you to proofread, edit, and track changes to your content using ChatGPT. … > Website: editgpt.app > Creator: @shuafeiwang.
editGPT: Proofread, edit and track changes to your content using …
Nov 11, 2024 … chat gpt actually gave a really good. system to pay off debt. the first one is to create a budget. it says that budget can help you. track your …
How can I effectively manage costs associated with AI tools?
Many people think that tracking AI costs is just about monitoring usage. I believe it’s much more nuanced. You can actually leverage price modeling to predict your expenses based on anticipated token usage.
For instance, analyzing historical data can help you set budget guidelines. This way, you avoid those nasty surprises when the bill arrives!
Most experts suggest using built-in analytics tools, but I think creating custom dashboards tailored to your specific needs is far more effective. This approach empowers teams to track their own consumption and optimize accordingly.
According to Dan Holloran from New Relic, “Real-time monitoring of OpenAI application usage allows companies to manage costs effectively while ensuring robust performance.” That’s a solid point!
Another angle is involving finance and tech teams in discussions about token usage. This collaboration can provide a holistic view of costs and help in crafting better budget forecasts.
Lastly, consider the financial implications of scaling your AI usage. Understanding how increased usage correlates with your financial returns can inform smarter investment decisions.
What is GPT tracking and why is it important?
Many folks think GPT tracking is just about monitoring usage. I believe it’s way more than that. It’s all about understanding how your AI interacts with users and optimizing that experience.
Real-time tracking gives insights into performance, helping you spot issues before they become problems. For example, you can analyze response times and tweak your model accordingly. According to Dan Holloran from New Relic, “Real-time monitoring of OpenAI application usage allows companies to manage costs effectively while ensuring robust performance.”
Some might argue that basic metrics are enough. I think customizing your analytics is the way to go. Collaborating with data scientists to create tailored dashboards can provide deeper insights. This approach empowers teams to make data-driven decisions that enhance user satisfaction.
New topics like Financial Implications of AI Scaling should be explored. Understanding how scaling AI affects your budget is essential for long-term success. It’s not just about tracking; it’s about making informed financial choices.
What metrics should I focus on for user engagement?
Most people think user engagement metrics revolve around basic stats like clicks and views. I believe it’s deeper than that. Focus on interaction rates and session duration. These tell a richer story about user behavior.
Engagement isn’t just numbers; it’s about understanding user satisfaction. Feedback ratings are gold. They reveal how well your AI meets user needs. According to Dan Holloran from New Relic, “User engagement metrics are integral in informing the adjustments needed for optimal AI performance.”
Another angle? Look at how users interact with your AI outputs. Tracking preferences can guide future improvements. It’s that simple. I think leveraging qualitative data from user interactions is often overlooked.
Most companies rely on standard KPIs, but I feel innovative metrics can drive better insights. For instance, integrating gamification elements can boost engagement significantly. Gamification strategies, like rewards or challenges, can make interactions more enjoyable.
Lastly, consider behavioral analytics. Analyzing user patterns can lead to tailored experiences that keep users coming back. This is often neglected but can dramatically influence your AI’s success.
How do token pricing models affect my AI budget?
Token pricing models can seriously impact your AI budget. Each model from OpenAI has its own cost structure based on token usage. If you’re not careful, costs can spiral out of control.
Many believe that simply understanding the basic token pricing is enough. But I think digging deeper is crucial. Knowing how requests translate into costs can help you make better financial decisions.
For instance, if you monitor token usage effectively, you can align your budget with projected usage patterns. This proactive approach can prevent unexpected expenses. According to Dan Holloran from New Relic, “Being informed about token pricing can help organizations make strategic choices to control costs while deploying AI solutions.”
Collaborative budgeting is another angle. Involving finance and tech teams can provide a rounded understanding of expenses. Regular reviews of token usage reports can help mitigate risks associated with unexpected costs.
Ultimately, understanding token pricing isn’t just about keeping track; it’s about strategizing for the future. As AI usage scales, so should your financial strategies.
What are some best practices for enhancing AI performance?
Most people think tracking AI performance is all about numbers. I believe it’s about understanding how users interact with the AI. Real-time monitoring isn’t just for performance; it’s about creating a feedback loop that informs future improvements.
Many experts suggest focusing solely on predefined metrics. But I think customizing metrics based on specific user interactions can yield better insights. This way, teams can adapt models to meet actual user needs.
Engaging users for feedback is often overlooked. I think incorporating user input during the development phase can refine AI outputs significantly. This creates a product that resonates more with users.
As Dan Holloran from New Relic states, “Real-time monitoring of OpenAI application usage allows companies to manage costs effectively while ensuring robust performance.” This highlights the dual benefit of monitoring: performance and cost management.
Another great approach is collaborative model training. Instead of just relying on historical data, why not involve users in the training process? Their real-world experiences can shape model evolution.
Finally, understanding user engagement metrics can’t be ignored. According to Louis Leung from New Relic, “Analyzing response metrics allows organizations to understand their model’s strengths and weaknesses.” This insight is golden for optimizing AI performance.
Real-time monitoring is a game changer for AI performance. It gives companies a peek into how their applications are doing at any moment. This means you can catch issues before they snowball.
Most folks think that tracking basic metrics is enough. But I believe in digging deeper—user behavior insights can reveal hidden patterns. This approach can lead to smarter adjustments and better user experiences.
According to Dan Holloran from New Relic, “Real-time monitoring of OpenAI application usage allows companies to manage costs effectively while ensuring robust performance.” This insight is gold for anyone serious about optimizing AI.
Consider collaborative methods for gathering user feedback. Involving users in the feedback loop not only improves performance but also builds trust. It’s about creating a partnership with your audience.
Let’s not forget the financial implications of scaling AI usage. Understanding how AI impacts your budget is key. This can help steer your decisions and maximize returns.
Most folks think user engagement metrics are just numbers on a dashboard. I believe they’re the heartbeat of AI applications because they reveal how users truly interact with the tech. Metrics like session duration and feedback ratings tell a story about user satisfaction.
Engaging users in feedback loops can be a game changer. It’s not just about functionality; it’s about how well the AI resonates with its audience. According to Dan Holloran from New Relic, “User engagement metrics are integral in informing the adjustments needed for optimal AI performance.”
Going beyond standard metrics, let’s talk about the potential of gamification. Instead of just tracking interactions, why not make it fun? Adding rewards and challenges can skyrocket user engagement.
Most people think token pricing is straightforward. I believe it’s complex because different models have unique structures that can surprise you.
Understanding how tokens translate into costs can save you from unexpected bills. According to Dan Holloran from New Relic, “Being informed about token pricing can help organizations make strategic choices to control costs while deploying AI solutions.”
Collaborative discussions between finance and tech teams can reveal hidden insights. This approach ensures everyone is on the same page about costs and usage.
Let’s not ignore the financial implications of scaling AI. As usage grows, so do costs, and aligning budgets with projected needs is key.
Most companies think they can just throw money at AI tools and hope for the best. I believe that smart cost management is key. By forecasting token usage, you can avoid those nasty surprises on your bill.
Tracking real-time metrics is a game changer. It lets you see where your money is going and adjust accordingly. As Dan Holloran from New Relic puts it, “Real-time monitoring of OpenAI application usage allows companies to manage costs effectively while ensuring robust performance.”
Don’t just rely on generic strategies. Create tailored approaches that fit your specific needs. Collaborating with finance and tech teams can unveil insights that save you a fortune.
Another angle? Implement budgetary guidelines based on historical usage data. This way, you’re not just reacting to costs; you’re proactively managing them.
Real-time observability is a game changer for AI applications. It offers insights that help identify issues before they frustrate users. With observability, businesses can maintain high service quality.
Many experts think logging is enough. I believe it’s about proactive monitoring. You need to anticipate user needs and adjust accordingly.
According to Louis Leung from New Relic, “Adopting observability measures ensures that every transaction provides meaningful insights into user behavior.” This is spot on! User behavior data drives better service delivery.
Consider A/B testing as an alternative. Comparing different model versions can reveal what users truly prefer. It’s a direct way to enhance user engagement.
The role of user feedback is often underestimated. It’s essential for continuous improvement. Engaging users can lead to innovative solutions and better AI performance.
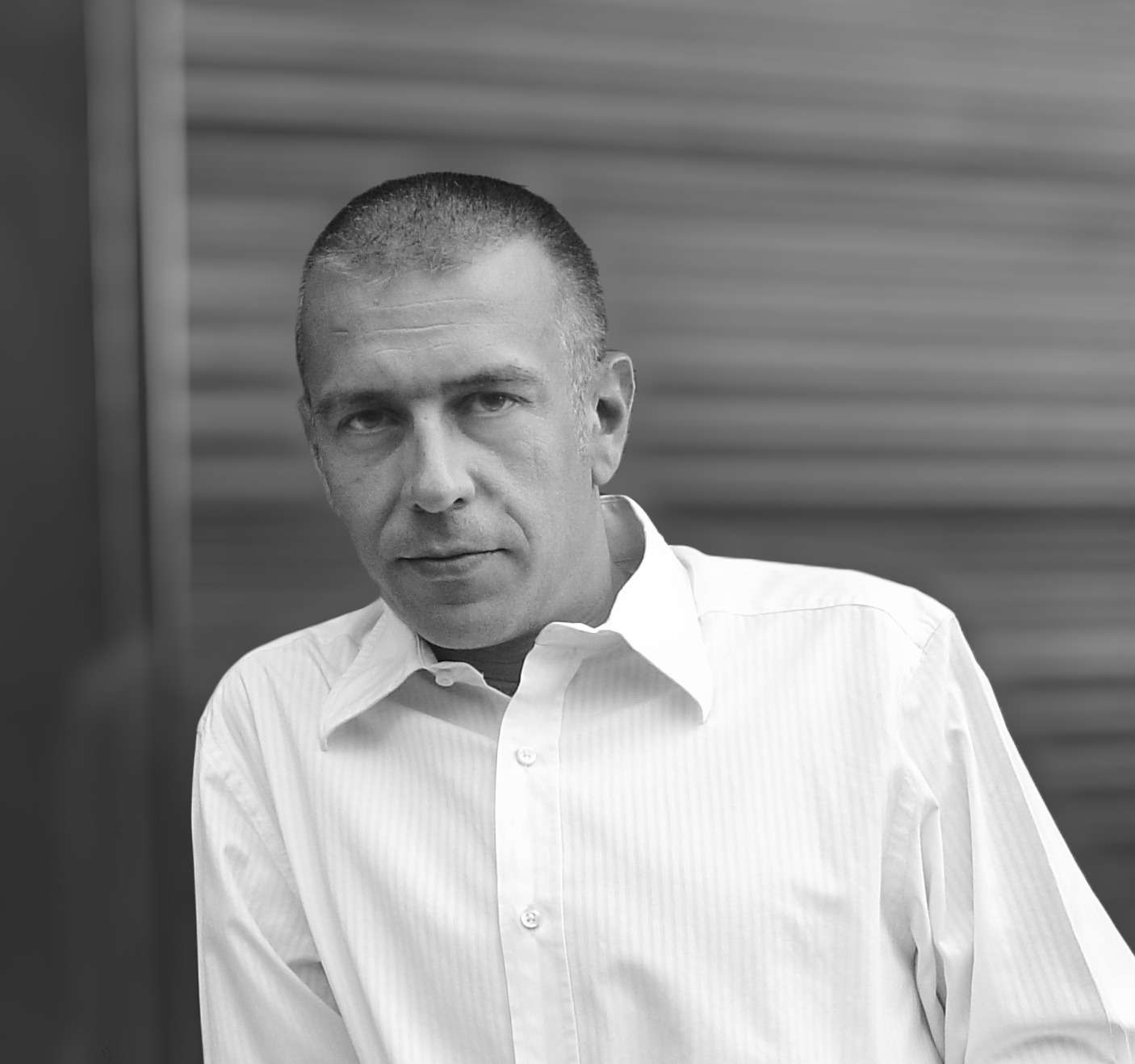
Albert Mora is an internationally renowned expert in SEO and online marketing, whose visionary leadership has been instrumental in positioning Aitobloggingas a leader in the industry.